Process Variation in Lean Six Sigma. Everything to Know
Process variation is one of the most common obstacles I encounter that prevents organizations from reaching their potential.
At its core, process variation refers to uncontrolled or unexpected differences in a process’s outputs. While some degree of natural variability exists in all processes, excessive variation is the enemy of efficient, high-quality operations.
Master Process Variation Control with Six Sigma Green Belt Certification
Become a catalyst for continuous improvement and excellence
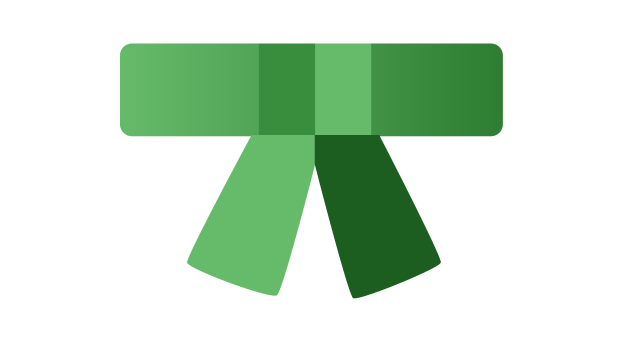
With years of experience applying continuous improvement methodologies across manufacturing, healthcare, and financial services, I’ve seen firsthand the impact process variation can have on quality, efficiency, and costs.
Key Highlights
- Defining process variation – Common vs special cause and the impact on consistency
- Major sources of variation – Raw inputs, methods, environment, people
- Types of output variability – Dimensions, defects, cycle times
- Core metrics for analyzing variability – Standard deviation, control limits, CpK
- Methods to reduce variation – SPC, standard work, mistake proofing and more
- Building quality at the source – Error prevention over inspection
- Leveraging experiments to optimize processes
- Sustaining gains from Six Sigma projects long-term
This article covers the sources and types of process variation based on my expertise with statistical process control and Lean Six Sigma principles.
I’ll also provide proven methods for analyzing, controlling, and reducing variation to transform business performance.
Whether you’re struggling with inconsistent quality, delays, or rising costs, understanding and managing process variation is essential. I’ll share field-tested tips for leveraging Six Sigma metrics, experimentation, and mistake-proofing to sustainably improve any business process.
By the end, you’ll understand why getting process variation under control provides the foundation for efficient, smooth-flowing operations and top-tier products and services.
Let’s dive in!
Defining Process Variation
As a foundation, process variation refers to uncontrolled or unexpected differences in the output of a business process.
While some degree of natural variability exists in all processes, excessive variation negatively impacts quality, productivity, and consistency.
Let’s explore the main types and causes:
Sources of Process Variation
Based on decades of applying statistical process control across various industries, I’ve categorized process variation into four primary sources.
Common Cause Variation
Common cause variation represents the natural or random variability inherent in any process over time.
Even with stable inputs and methods, some differences in outputs are expected from normal fluctuation. Changes within common cause systems happen frequently but have minor effects.
Special Cause Variation
Special cause variation refers to unusual or unexpected changes traced back to specific circumstances.
Examples include raw material problems, operator errors, software glitches, equipment failures – anything well outside the norm for that process. Special causes lead to much more drastic deviations.
Assignable Variation
I classify assignable variation as preventable variability resulting from issues like substandard inputs, maintenance problems, poor process design, inadequate operator skills, and ineffective supervision. Thorough analysis can identify and address these assignment causes.
Random Variability
Finally, random variability comes from natural variation even in stable processes with all controls in place. Chance variations in environment, materials, and durations fall under this source.
Random variation cannot be eliminated, but it can be accounted for and minimized.
Understanding these core sources of variation provides clues as to where to start analyzing and controlling variability.
Types of Process Variation
Now that we’ve explored the common sources leading to uncontrolled variation, let’s examine some of the most prevalent ways variation manifests in business processes…
Variability in Outputs
The outputs of any process ultimately impact the customer experience and must align with critical quality specifications. Excess variation in outputs directly reduces product performance and reliability.
Common examples include:
Dimensional Variation
In manufacturing, variability in the physical dimensions of products falls outside tolerance limits resulting in quality issues. Production processes struggle with consistency in factors like length, weight, straightness, and more.
Defect Rates
Defect rates refer to the frequency of products or transactions containing flaws, errors, or quality issues per total output. High or inconsistent defect rates hint at problems with process stability.
Cycle Times
The time needed to complete key process steps or the overall cycle from order to delivery demonstrates variation if durations fall outside expected ranges. Excess variability in cycle times hinders scheduling and on-time performance.
Variability in Inputs
Process inputs, including materials, environment conditions, information flows, equipment, and resource environmental impact output consistency.
Raw Material Properties
Physical product components introduce variability through differences in size, purity, mechanical specs, and more. Screening and testing help, but some fluctuation persists.
Environmental Conditions
Temperature, humidity, lighting, and contamination levels alter process dynamics. Environmental variability contributes to output inconsistency even with stable methods.
Measurement System Variation
Even input measurement tools have variability that gets propagated through processes. Calibration and gage R&R confirmation help mitigate, but 100% elimination proves difficult.
Understanding how variation alters both process inputs and outputs builds context to strategically address problems with capability analysis and experimentation.
Managing Process Variation
Once the sources and types of variability are understood, a combination of statistical, systems, and cultural enablers allows driving toward stability.
Getting variability under control requires a multi-pronged approach combining statistics, systems thinking, and culture transformation.
Here are proven techniques:
Statistical Process Control
Statistical methods quantitatively analyze variation while providing signals when processes go out of control:
Control Charts
Control charts plot output data over time, calculating average and upper/lower control limits based on historical volatility.
Points outside the limits indicate special cause variation requiring investigation. Control charts help teams differentiate common vs special cause variation.
Capability Analysis
Short-term and long-term capability ratios compare output distribution spreads against specification limits revealing stability issues.
A process with indices under 1.0 likely has excessive variation and risks not meeting customer requirements.
Together, these statistical techniques diagnose if and when variation exceeds expected levels across the distinct phases of a process. This prevents problems from accumulating downstream.
Error Proofing
Error proofing introduces physical and digital constraints to prevent defects by making improper conditions impossible:
Poka-Yoke Devices
Poka-Yoke devices foolproof sensors, guides, gauges, and alerts enable processes to automatically detect or avoid potential failure modes in real-time.
The goal is to eliminate conditions causing variation at the source rather than just inspect quality at the end.
Visual Controls
Visual indicators, escalation signals, metrics boards, and status displays promote rapid awareness of any abnormal issues arising on the frontline. Variation is instantly flagged to drive local response.
Standardized Work
Documenting and continually improving standardized work procedures, best practices, and work instructions reduces uncontrolled variation introduced by operators.
Adherence and compliance are key.
Continuous Improvement
Finally, developing a culture focused on sustaining variability gains through incremental optimization sustains top-tier performance:
Root Cause Analysis
Statistical tools like the Five Whys methodology track down the origin of problems to address special causes and prevent a recurrence.
Experimentation
Design of experiment techniques empowers teams to systematically adjust inputs to derive correlations and empirically optimize settings. This prevents guessing.
Optimization
Incremental testing and refinement of inputs, methods, and parameters tunes performance on key output metrics like defect rates and cycle times.
Rome wasn’t built in a day and variability won’t be eliminated overnight. But through systems thinking, analytics, and committed leadership, creating stability delivers breakthrough results.
Discover the Power of Lean Six Sigma Methodologies
Learn cutting-edge techniques to eliminate process waste with Lean Six Sigma Overview.
Benefits of Controlling Process Variation
In my extensive experience guiding organizations on their operational excellence journey, transforming processes plagued by variation delivers tangible business results.
Once leadership teams see the performance lift in metrics directly tied to profits, productivity, and customer satisfaction, they become fully invested in capability enhancement and variation reduction.
While foundational to quality management, lowering variability positively impacts multiple facets beyond just product and service consistency.
Let’s examine some of the key benefits I’ve measured across industries after applying the techniques detailed in this guide:
Improved Quality
The most intuitive gain coming from statistical control of a process is enhanced output predictability and conformance to specifications:
More Consistent Outputs
Cycle time variation contains a process predicting throughput. Defect rate variation causes product recalls. In contrast, minimal variation sustains specifications without reactionary changes.
I’ve helped teams reduce dimension variation by 60%, slash late deliveries by 45%, and improve test precision 7-fold.
Reduced Defects
Variation causes defects by allowing abnormal, out-of-spec conditions to arise. Mistake-proofing product flows contain this risk.
For a medical lab, we piloted changes cutting erroneous result rates from 1.2% to under 0.4% in 6 weeks through fail-safes and alerts
Increased Efficiency
A smoother, steadier workflow prevents disruptions that throttle productivity and responsiveness:
Reduced Waste
Too much variation leads to costly scrap and rework when problems surface downstream.
Optimizing a steel producer’s process raised yield by $125K annually through DOE and tighter controls.
Faster Cycle Times
Lower variation enables seamless workflow and rapid throughput by eliminating stops and slowdowns.
A client boosted on-time delivery by 11% after reducing special causes and streamlining changeovers.
Improved Throughput
Capable, predictable processes sustainably operate at near-optimal rates. A packaging line we worked with increased monthly volume shipped by 5.2% through steadier uptime and faster speeds.
Lower Costs
Finally, increased profits result from less firefighting, inspection, and duplicated effort.
Less Rework
Scrap rates and repair work plunge when machines and methods stay in control. One manufacturer achieved 9% cost savings by curbing deviations requiring reprocessing.
Fewer Unexpected Failures
Unplanned downtime events become rare through source prevention and controls. This allowed an automotive supplier to cut line failures by 44% and save $3.1M annually.
Parting Notes
After 20+ years of practicing continuous improvement, I’m still energized and passionate about helping organizations achieve operational excellence and managing process variation that sits right at the heart of that mission.
I hope this guide has helped you why variability acts as the silent enemy of quality and efficiency.
The techniques I’ve shared represent a sampling of proven methods for analyzing and controlling variation. Master these and you hold the key to unlocking substantial performance gains.
Yet, transforming processes plagued by variability requires more than just statistics and systems thinking. Leaders must drive culture change at all levels to recognize and respond to abnormal conditions quickly.
Operators have to rigorously comply with validated standards. Everyone needs to focus on mistake-proofing.
Sustaining the benefits also takes persistence through control plans and ongoing metrics monitoring, optimization, and training. But for teams ready to instill that kind of disciplined excellence into their DNA, the sky’s the limit.
Take that first step now by running a pilot process improvement project in a small controlled setting.
Let the compelling results speak for themselves on potential scaleup.
SixSigma.us offers both Live Virtual classes as well as Online Self-Paced training. Most option includes access to the same great Master Black Belt instructors that teach our World Class in-person sessions. Sign-up today!
Virtual Classroom Training Programs Self-Paced Online Training Programs