Attribute Agreement Analysis in Lean Six Sigma. Everything to Know
Data is key to making informed and successful decisions. Attribute agreement analysis steps in as an indispensable tool.
This analysis is crucial in fields like manufacturing, healthcare, and services, where human evaluation plays a big role in quality control.
Ensure Data Integrity with Advanced Analysis
Is your data reliable? With Lean Six Sigma Green Belt Certification learn to ensure data accuracy and make confident, data-driven decisions.
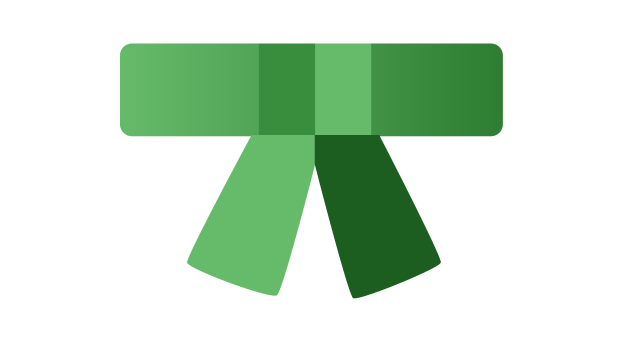
Pinpointing and addressing the sources of variation in those assessments helps improve products and services, cut down on issues, and boost overall efficiency.
It evaluates the repetitiveness and reproducibility of data attributes like pass/fail, go/no-go, and so on. This provides the confidence to make informed and data-driven decisions that are grounded in accurate, reliable information, not just subjective biases.
Key Highlights
- Attribute agreement analysis is an essential tool for evaluating the consistency and accuracy of measurement systems involving human judgment or subjective assessments.
- It determines the level of agreement among appraisers or inspectors when classifying attributes like pass/fail, go/no-go, or conform/nonconform.
- This analysis is crucial for ensuring reliable data and decision-making in industries like manufacturing, healthcare, and services.
- By identifying and addressing sources of measurement variation, attribute agreement analysis leads to improved product/service quality and reduced costs from non-conformities.
- It involves calculating agreement statistics like percentage of agreement, kappa values, and confidence intervals to quantify appraiser consensus.
- Proper training, guidelines, and the right tools are essential for conducting and interpreting this analysis effectively.
- When leveraged correctly, attribute agreement analysis provides the insights needed to drive continual quality improvements across the organization.
What is Attribute Agreement Analysis?
Attribute agreement analysis is a statistical tool to evaluate the consistency and reproducibility of measurement systems.
It achieves this by quantifying the level of agreement among diverse appraisers, this analysis ensures the data you’re collecting is reliable and consistent enough to make truly informed decisions.
The key purpose is to measure the repeatability and reproducibility of your attribute measurement system. This helps you identify and address potential sources of variation from appraiser subjectivity or bias.
Ultimately, attribute agreement analysis enables consistent decision-making when accepting or rejecting products and processes based on those attribute inspections.
No more worrying about unreliable data skewing your choices.
It’s a game-changing way to drive quality and efficiency by basing decisions on a shared, trustworthy foundation.
Sure, proper training and the right tools are a must to get the most out of this analysis. But when leveraged correctly, it unlocks invaluable insights to continually improve your operations.
When to use attribute agreement analysis?
Attribute agreement analysis should be performed whenever there is a need to validate a measurement system involving human judgment. Some scenarios where it is commonly used include:
- New measurement system studies during production line setup
- Periodic re-certification of existing measurement systems
- Comparison of multiple appraisers, instruments, or locations
- Attribute data collection for statistical process control (SPC)
- Situations where variable data cannot be obtained
Advantages and disadvantages
Advantages
- Helps reduce measurement errors from appraiser inconsistency
- Relatively simple to perform compared to variable studies
- Useful when defect classification is more practical than actual measurements
- Supports quality improvement by identifying training needs
Disadvantages
- Provides less precise information than variable studies
- Proper training of appraisers is critical for reliable results
- Sample size requirements can be higher than variable studies
- Unable to detect small process shifts effectively
By evaluating the degree of agreement in attribute classification, this analysis is a valuable tool for ensuring the integrity of quality data, especially in situations where human judgment is involved.
Steps in Attribute Agreement Analysis
Data Collection Requirements
Attribute agreement analysis requires specific data to be collected in a structured manner. The first step is to identify the attribute or characteristic being evaluated.
This could be a pass/fail criterion, a go/no-go judgment, or a conform/non-conform assessment. It’s essential to clearly define the attribute and the criteria for each possible outcome.
Next, you need to determine the number of appraisers or inspectors who will evaluate the samples. Typically, at least two appraisers are required, but more can be included to assess consistency across multiple individuals.
The samples to be evaluated should be representative of the population or process being studied. The sample size should be large enough to provide statistically significant results, and it’s often recommended to have at least 30 samples.
During data collection, each appraiser independently evaluates each sample and records their assessment (e.g., pass or fail) for the attribute being studied.
It’s crucial to ensure that the appraisers are properly trained and understand the evaluation criteria to minimize subjective biases.
Calculating Agreement Statistics
Once the data is collected, various agreement statistics can be calculated to quantify the level of agreement among the appraisers. Some common statistics used in attribute agreement analysis include:
- Percentage Agreement: This is the simplest measure and represents the proportion of samples for which all appraisers agreed on the assessment.
- Fleiss’ Kappa: This statistic adjusts for the agreement that would be expected by chance and provides a measure of the true agreement beyond random chance.
- Cohen’s Kappa: Similar to Fleiss’ Kappa, but used when there are only two appraisers or when evaluating the agreement between pairs of appraisers.
- Kendall’s Coefficient of Concordance: This statistic measures the overall agreement among multiple appraisers when there are more than two possible outcomes (e.g., pass, fail, marginal).
These agreement statistics can be calculated manually using formulas or with the help of statistical software or online calculators.
Interpreting Results
After calculating the agreement statistics, the next step is to interpret the results and determine if the level of agreement is acceptable.
This typically involves comparing the calculated values to established guidelines or industry standards.
For example, a common guideline for attribute agreement analysis suggests that a Fleiss’ Kappa value above 0.75 indicates excellent agreement, while a value below 0.4 indicates poor agreement and may require corrective actions.
If the agreement levels are deemed unacceptable, potential causes should be investigated. This may include factors such as inadequate appraiser training, ambiguous evaluation criteria, or inherent variability in the process or product being evaluated.
Based on the interpretation of the results, appropriate actions can be taken. These may include retraining appraisers, refining evaluation criteria, or implementing process improvements to reduce variability.
It’s important to document the attribute agreement analysis process, including the data collected, calculations performed, and any corrective actions taken. This documentation serves as a record and can be used for future reference or auditing purposes.
Tools and Software for Attribute Agreement Analysis
There are several tools and software available to perform attribute agreement analysis efficiently. These range from simple spreadsheet templates to dedicated statistical software packages.
The choice depends on the complexity of the study, the sample size, and the user’s familiarity with the tool.
Excel templates
Microsoft Excel is a widely accessible option for conducting attribute agreement analysis, especially for small to medium-sized studies.
Several pre-built templates are available online that can calculate agreement statistics like Fleiss’ kappa, multi-rater kappa, and percent agreement.
These templates typically require users to input their data and the formulas automatically compute the results.
While convenient for basic analyses, Excel templates may have limitations in handling larger datasets or providing advanced analytical capabilities.
Statistical software like Minitab
Dedicated statistical software like Minitab offers robust tools for attribute agreement analysis.
Minitab’s measurement system analysis module includes functions for attribute agreement analysis, such as calculating kappa statistics, conducting hypothesis tests, and generating detailed reports.
These software packages are particularly useful for larger studies, complex analyses, or when integrating attribute agreement analysis into a broader quality control program.
However, they often require specialized training and can be more expensive than spreadsheet-based solutions.
Online calculators
Several online calculators are available that can perform attribute agreement analysis with minimal setup.
These web-based tools typically require users to input their data and select the appropriate analysis method.
The calculator then computes the agreement statistics and provides interpretations. Online calculators are convenient for quick analyses or when users do not have access to dedicated software.
However, they may have limited functionality compared to comprehensive statistical packages, and data security should be considered when using online tools.
Regardless of the tool or software chosen, it is crucial to understand the underlying assumptions, limitations, and interpretation guidelines for attribute agreement analysis.
Proper training and adherence to best practices are essential to ensure reliable and meaningful results.
Best Practices and Guidelines
Following best practices and guidelines is crucial for obtaining accurate and reliable results when conducting an attribute agreement analysis. Here are some key areas to focus on:
Sample Size Determination
The sample size used has a direct impact on the precision of the agreement statistics calculated.
In general, a larger sample size will provide more reliable estimates of agreement. However, there are practical constraints like cost, time, and availability of samples that need to be considered.
Several factors influence the appropriate sample size, including the desired confidence level, the anticipated level of agreement, the number of appraisers, and the acceptance criteria being used.
Statistical techniques like power analysis can help determine the minimum sample size required to detect a specified level of disagreement with adequate confidence.
Some general guidelines are to use at least 50-100 samples when evaluating a single appraiser against a standard, and 300 or more samples when evaluating agreement among multiple appraisers.
However, these are just rules of thumb – proper sample size analysis is recommended.
Appraiser Training
The skill and consistency of the appraisers evaluating the samples are critical.
Thorough training on the measurement process, specifications, and evaluation criteria is essential before conducting an agreement analysis study. This training helps minimize appraiser bias and inconsistencies.
It is best practice to use pilot studies and qualification tests to ensure appraisers perform at an acceptable level before the full study.
Ongoing monitoring, retraining when needed, and rotating appraiser roles can further improve consistency.
Documentation
Detailed documentation of the entire attribute agreement analysis process is a must.
This includes specifying the product/process characteristics being evaluated, the sampling plan, appraiser details, decision criteria, data collection methods, and analysis procedures.
Clear documentation ensures the study can be recreated if needed and allows for a proper review of the results.
It also serves as a reference for future similar studies. Maintaining revision control on documentation is highly recommended, especially in regulated industries.
Applications of Attribute Agreement Analysis
Attribute agreement analysis is a powerful tool that finds widespread application across various industries and methodologies focused on quality improvement and process optimization. Here are some key areas where it is commonly employed:
Quality control
In quality control, attribute agreement analysis is crucial in ensuring that measurement systems and inspection processes are reliable and consistent.
It helps identify potential sources of variation among appraisers or inspectors when assessing product or service attributes.
By quantifying the level of agreement, organizations can take necessary actions to improve training, clarify operational definitions, or refine measurement procedures, ultimately enhancing the quality of their products or services.
Six Sigma
Six Sigma, a data-driven methodology for process improvement, heavily relies on attribute agreement analysis as part of its measurement system analysis (MSA) toolkit.
In Six Sigma projects, attribute agreement analysis is used to validate the capability of measurement systems involving human appraisers or inspectors.
It helps determine if the measurement process is acceptable for its intended use, ensuring that the data collected is reliable and suitable for making informed decisions about process improvements.
Lean manufacturing
Lean manufacturing principles emphasize the elimination of waste and continuous improvement.
In this context, attribute agreement analysis is valuable for assessing the consistency and reliability of visual inspection processes, which are common in many lean environments.
By identifying and addressing disagreements among inspectors, organizations can streamline their processes, reduce defects, and improve overall efficiency and quality.
Case studies
Numerous case studies across various industries have demonstrated the practical applications and benefits of attribute agreement analysis.
For example, in the automotive industry, it has been used to evaluate the consistency of vehicle inspections, leading to improved training programs and standardized inspection procedures.
In the healthcare sector, it has been employed to assess the agreement among medical professionals in diagnosing conditions or interpreting test results, contributing to better patient outcomes and quality of care.
These applications highlight the versatility and importance of attribute agreement analysis in ensuring consistent and reliable measurement systems, ultimately driving quality improvement, process optimization, and operational excellence across diverse industries and methodologies.
Comparison with Other Agreement Analyses
Variable Agreement Analysis
Attribute agreement analysis is often compared to variable agreement analysis, which is another method used in measurement system analysis (MSA).
Variable agreement analysis is used when the measured data, such as length, weight, or temperature, is continuous. In contrast, attribute agreement analysis is used when the data is binary or categorical, such as pass/fail, go/no-go, or good/bad.
The main difference lies in the way the data is analyzed and the statistics used. Variable agreement analysis typically uses metrics such as percent study variation (%SV), percent gage repeatability and reproducibility (%GRR), and intraclass correlation coefficient (ICC).
On the other hand, attribute agreement analysis uses statistics like confidence intervals, kappa values, and attribute agreement analysis charts.
Another key distinction is that variable agreement analysis accounts for both bias and variability in the measurement system, while attribute agreement analysis focuses solely on variability or disagreement between appraisers.
Analytic vs Attribute Methods
Attribute agreement analysis falls under the category of attribute measurement system analysis (MSA) methods, which evaluate the performance of measurement systems that produce binary or categorical data.
These methods are contrasted with analytic MSA methods, that are used for continuous data.
Analytic methods, such as variable agreement analysis, gage repeatability and reproducibility (GRR) studies, and analysis of variance (ANOVA), rely on quantitative measurements and provide numerical estimates of measurement system variability.
They are generally more complex and require more data than attribute methods.
Attribute methods, on the other hand, are simpler and require less data. They are based on counts or frequencies of agreement or disagreement between appraisers or between an appraiser and a known standard.
Attribute agreement analysis is one of the most commonly used attribute MSA methods, along with attribute gage repeatability and reproducibility (GRR) studies and attribute gage studies.
The choice between analytic and attribute methods depends on the type of data being measured and the specific requirements of the measurement system evaluation.
Analytic methods are preferred when precise quantitative measurements are needed, while attribute methods are suitable for pass/fail or categorical data.
SixSigma.us offers both Live Virtual classes as well as Online Self-Paced training. Most option includes access to the same great Master Black Belt instructors that teach our World Class in-person sessions. Sign-up today!
Virtual Classroom Training Programs Self-Paced Online Training Programs