Statistical Process Control – Specification Limits. Everything to Know
In quality management and workflow refinement, specification limits play a huge role in defining normalcy’s bounds.
They represent client demands, ensuring offerings meet use and satisfy needs.
Understanding and properly leveraging specification limits becomes key for businesses aiming at steadiness, less deviation, and stronger client loyalty.
This guide deeply explores specification limits’ complexities, how they link with tolerance borders, and their role within Statistical Process Control’s (SPC) framework.
We’ll learn about under-control versus over-control methods, the Taguchi Loss Function’s concepts, plus strategies for optimizing and surveillance.
Also, find case studies and proven practices to equip you to handle specifics skillfully within operations.
Key Highlights
- Specification limits lay tolerable variation for product/process attributes, confirming satisfaction of client needs.
- Understanding specification limits prove pivotal for proficient process guidance and ability analysis.
- Spec boundaries diverge from monitoring control limits detecting stability changes with time.
- Methods classify as under-control or over-control contingent on performance against specification limits.
- Taguchi’s Loss Function sizes variation’s expenses, informing processes’ centeredness inside spec allowances.
- Statistical Process Control (SPC) combines specification limits with charts and capability metrics for complete workflow tracking toward refinement.
- Employing a sturdy specification limits plan synchronizes making with client outlooks, cultivating quality and satisfaction propelling enterprises amid fluid surroundings.
What are Specification Limits?
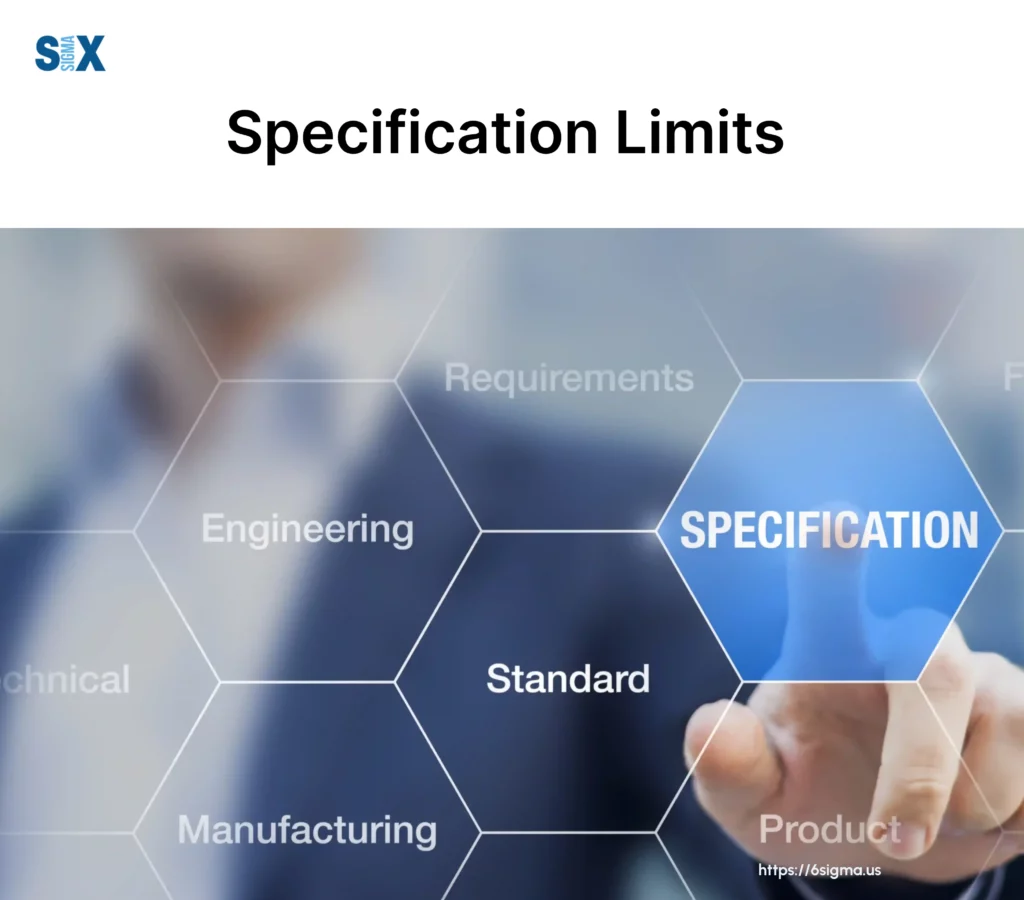
Specification limits mark the acceptable extent of variability for a product or process attribute.
They represent max/min values needed for meeting client needs and desires. Limits generally arise from designers, industry guidelines, or buyer specifications.
In quality guidance and refinement, specification limits play a pivotal part in judging if making methods reliably stay inside wanted regions.
Within spec boundaries signals capable behavior and conforming outputs. Outside flags are incapable of finding potential defects.
Sources determining spec borders include:
- Buyer demands, satisfying particular wants
- Industry norms maintaining homogeneity, safety, and interconnectivity
- Design stages outlining mandatory specs based on intended use, material traits, and other engineering considerations
Importantly, specification limits contrast control limits used within Statistical Process Control to surveil and manage inherent change over time.
Understanding specification limits proves vital for guaranteeing quality, customer trust, and meeting sector standards legally and ethically. Tracking and containing variation optimizes outcomes and reduces risky departures from specifications.
The journey continues toward output mastery through meticulous oversight, flexibility refining parameters in rhythm with evolving markets, and propelling enterprises equipped to enhance client relations.
Specification Limits vs. Control Limits
It’s important to understand the distinction between specification limits and control limits when it comes to statistical process control and process capability.
Specification limits define the boundaries that separate acceptable product or process performance from unacceptable. They are set by either the customer requirements, industry standards, safety considerations, or prior process knowledge.
Control limits, on the other hand, are different statistical limits used for monitoring the stability of a process over time using control charts.
Control limits are calculated from the inherent variation in the process itself, usually at +/- 3 sigma from the mean of the data.
Processes operating within the control limits are considered stable and “in control”, while points plotting outside the limits may indicate an unstable or “out of control” condition requiring investigation and adjustment.
The key difference is that specification limits are fixed, externally set requirements, while control limits are statistically derived monitoring limits that indicate if the process is stable and predictable.
A process can be operating within its control limits but still not meet specification limits, which would indicate a capable but off-target process requiring adjustment.
Conversely, a process may be capable of meeting specifications but operating outside of its control limits, signaling an unstable process in need of improved control.
Having both specification limits and control limits allows you to assess process capability (can we meet requirements?) as well as process control (is the process stable and predictable?).
Using control charts along with process capability metrics gives you a comprehensive view of your quality situation. Proper statistical control is generally a prerequisite for accurate capability analysis against specification limits.
Under-control and Over-control Processes
An important concept related to specification limits is whether a process is under statistical control or not.
A process is considered under control when the variation present is consistent, predictable, and inherent to the process itself. This natural variation is caused by common causes that are essentially random and unavoidable.
When a process exhibits this state of being under control, it means the process is stable, with the variation being randomly distributed around the mean or target value within the calculated control limits.
The control limits, which are different from specification limits, indicate the bounds of normal variation for the given process.
On the other hand, an over-control process is one where the variation is artificially reduced beyond the natural variation through overcorrection or tampering.
This results in a distribution with less variation than expected, which can be undesirable as it indicates the process is being overadjusted frequently.
Properly determining if a process is under control or over control is crucial. An under-control process operating within the specification limits is the ideal state, producing consistent results that meet customer requirements.
However, an over-controlled process may seem desirable at first due to tighter variation, but it can lead to excessive costs from unnecessary adjustments and wasted effort.
The key statistical tools to assess if a process is under control include control charts and process capability metrics like the Cp and Cpk indices.
Control charts monitor process behavior over time, while capability metrics quantify how well the process variation fits within the specification limits.
Applying these tools properly allows distinguishing an ideal under-control process from an artificially over-controlled one.
Taguchi Loss Function and Process Targeting
The Taguchi loss function provides a way to measure the loss or cost to society from producing products that fail to perform as intended.
Even if a product meets specification limits, any deviation from the target performance characteristic results in some loss to the customer or producer. The Taguchi loss function quantifies this loss.
The fundamental principle is that quality is the avoidance of variation from a nominal or target value, rather than simply meeting specification limits.
Products that deviate from the target value, even if within specifications, lead to some loss of quality. The loss increases quadratically as the deviation from the target increases.
By setting specifications tightly around the target value and reducing variation, producers can minimize the losses experienced by customers using their products.
This is the essence of process targeting – aiming the process means at the target value and minimizing variation around that value.
Effective process targeting requires:
- Identifying the target or nominal value for the key quality characteristic(s)
- Estimating the Taguchi loss function parameters
- Adjusting the process means hitting the target value
- Reducing common cause variation through continuous improvement
Control charts monitor the stability of the adjusted process mean and variance over time. Capability indices like Cpk measure the ability of the process to operate within specifications relative to the target.
The goal is to have a centered process with Cpk > 1.33 or higher.
This provides a buffer between the process mean and the specification limits, minimizing losses from product performance deviating from the target value. Targeting perfection and reducing variation is the path to the lowest costs and highest quality.
Statistical Process Control Strategy with Specification Limits
Having an effective statistical process control strategy is crucial for ensuring your processes operate within specified limits and produce consistent, high-quality output. A robust SPC strategy involves several key components:
Process Monitoring
Continual monitoring of the process through control charts is essential. Control charts provide a visual representation of how the process is performing relative to the specified limits.
Both variable charts (e.g. X-bar and R) and attribute charts (e.g. p, np, c, u) may be utilized depending on the data type.
Control Chart Analysis with Specification Limits
Simply plotting data on a control chart is not enough. The charts must be analyzed and interpreted correctly using the Western Electric rules or other control chart rules. This allows you to detect signals that the process may be going out of statistical control.
Capability Analysis
Conducting process capability studies is important for assessing whether the process is capable of consistently meeting specification limits.
This involves calculating metrics like the process capability indices (Cp, Cpk, Pp, Ppk) and establishing whether the capability is acceptable.
Root Cause Analysis
When assignable causes of variation are detected through the control charts, disciplined root cause analysis should be performed to identify and eliminate the sources of excessive variation.
Process Adjustment with Specification Limits
If the process is found to be off-target or exhibiting excessive variation, adjustments, and corrective actions must be taken to re-center the process on the target value and reduce variability.
Continuous Improvement
SPC should drive continuous improvement efforts by establishing a data-driven system for monitoring, reducing variation, and improving process capability over time.
Applying Statistical Process Control with Specification Limits
To illustrate the concepts of specification limits, process capability, and statistical process control, let’s walk through a case study example.
Suppose you are a quality engineer at a manufacturing plant that produces rubber gaskets for automotive applications.
The critical dimension for these gaskets is the inner diameter, which has a specified tolerance range of 25.0 mm ± 0.5 mm. Any gaskets outside this specification range of 24.5 mm to 25.5 mm are considered defective and cannot be shipped to customers.
Your first step is to study the process capability by collecting data from the manufacturing process over an extended period. You record samples of 50 gasket diameters every 2 hours and calculate the sample mean, range, and standard deviation statistics.
After collecting data for 25 production runs, you can calculate the overall mean of 24.98 mm and the long-term sigma of 0.25 mm based on the average moving range.
This results in a process capability ratio Cp = 0.5/0.25 = 2.0 and a performance ratio Ppk = 1.6, indicating that the process is currently capable of operating within the specification limits at the +/- 4 sigma level.
However, you notice that the process mean of 24.98 mm is shifting closer to the lower specification limit over time. You create an X-bar and R-control chart and detect that the process mean has drifted by nearly 1.5 sigma from the target.
Investigating further, you discover that a coolant chiller is not operating properly, causing the injection molding process to run hotter during certain periods.
By making adjustments to the chiller system and implementing tighter preventive maintenance procedures, you can re-center the process around the target mean of 25.0 mm.
Continued monitoring with control charts allows you to quickly detect and correct any future shifts or drifts in the process.
Applying robust statistical process control methods has allowed your plant to consistently produce gaskets within specification limits, reduce rework and scrap, and maintain a high level of customer satisfaction.
The case study demonstrates the importance of quantifying process capability, monitoring with control charts, and making data-driven adjustments to ensure an in-control and capable process.
Best Practices and Recommendations
When working with specification limits and statistical process control, there are several best practices to keep in mind:
Establish Reasonable Specification Limits
Specification limits should be set based on customer requirements, process capability data, and any relevant industry standards or regulations. Overly tight limits can lead to excessive waste and adjustment efforts, while loose limits allow too much variation that impacts quality.
Monitor Process Performance Regularly
Implement a process monitoring plan using control charts to track key quality characteristics over time. This allows you to detect issues like process drift or unstable variation before many defective units are produced.
Calculate Appropriate Capability Indices
Make sure to calculate and monitor relevant process capability metrics like Cp, Cpk, Pp, and Ppk. These provide insight into how well the process can operate within the specification limits long-term and short-term.
Implement Process Control Rules
Define objective statistical rules for detecting non-random patterns of variation on control charts. This allows you to identify potentially assignable causes that require adjustment or investigation.
Consider the Voice of the Customer
Always tie your specification limits and quality goals back to meeting customer needs and requirements. The voice of the customer should drive your quality systems.
Utilize Process Adjustment Methodologies
Have a systematic methodology like PDCA or DMAIC to make data-driven process adjustments when monitoring indicates the process is drifting or becoming incapable of meeting specifications.
Invest in Continuous Improvement
View SPC and specification limits as part of an overarching continuous improvement program. Regularly re-evaluate specifications, control strategies, and opportunities for variation reduction.
SixSigma.us offers both Live Virtual classes as well as Online Self-Paced training. Most option includes access to the same great Master Black Belt instructors that teach our World Class in-person sessions. Sign-up today!
Virtual Classroom Training Programs Self-Paced Online Training Programs